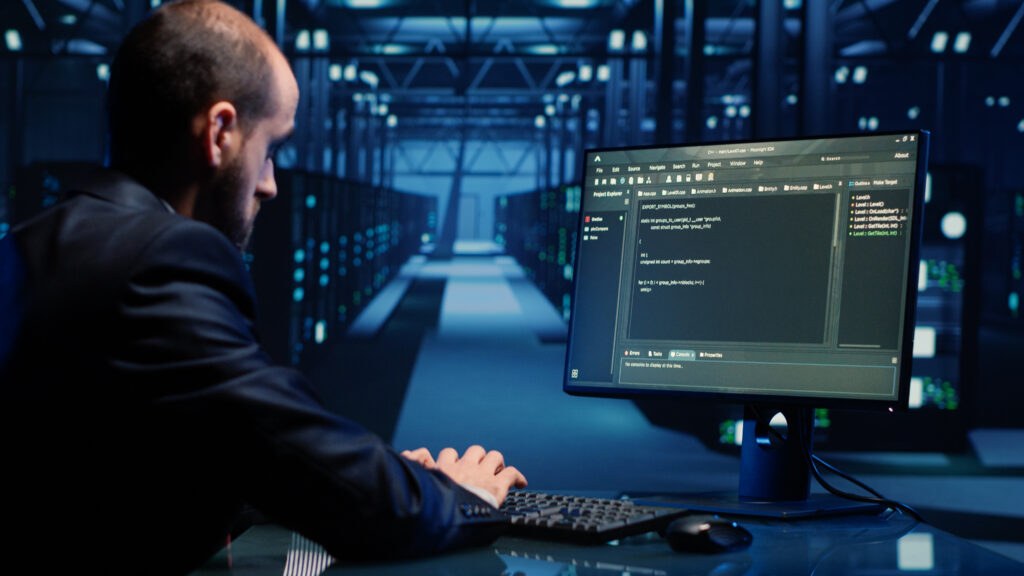
A lot has been said about how much pressure AI inflicts on data centers. These facilities are struggling to cover all the needs of AI, and the technology is just getting started. We are still in the very early days of AI’s development and it’s already demanding a lot of resources. So, a smarter approach is in order and a somewhat neglected so far approach could help – edge computing.
It seems that edge and AI could be a match made in heaven and the main beneficiary of that would be data centers. Edge AI seems like a perfect solution for many use cases, and it may also be of benefit for artificial intelligence as a whole.
First, a quick refresher. Edge computing refers to bringing a lot of computing infrastructure closer to the source or place where they are needed. For example, installing a server on a base station which covers the computing needs for the smart traffic lights in the area. Or having a server to crunch the numbers for a smart factory. The main idea of edge computing is to keep the main workload close to the place of needs but defer to data centers only for storage or more complex computing tasks. This way both the data center and the network will be less busy, which will save on resources while improving the service quality.
All of this makes it obvious that edge computing might just be an ideal solution for AI workloads. The 2023 Edge Advantage Report by NNT Data for example, shows that 70% of enterprises are using edge solutions to solve business challenges. And most experts agree that AI computing should happen close to where the data is generated, which means – at the edge.
How can Edge AI help
The idea of using edge computing for AI needs is so popular that it has already become its own niche. It’s called Edge AI and according to Data Center Knowledge, it means the processing of data when and where it’s generated, rather than relying solely on remote servers. So, the basics are the same as for regular edge computing, but the difference is that Edge AI is focused solely on AI workloads. This means that the edge servers are also more specific and tailored to the AI needs. Proponents of this approach say it will improve the operational efficiency of the companies.
“AI compute isn’t just the future, but it’s already here. Manufacturing enterprises, in particular, are moving AI systems out of the cloud and onto the edge, enabling faster data processing and reducing latency and cloud-server costs. Moving AI systems out of the cloud and onto the edge, particularly in manufacturing environments, results in faster processing of data from Internet of Things (IoT) devices and sensors, connected by local networks within factories. This reduces latency, cuts down on cloud-server costs, and opens up new possibilities for localized innovation,” says Kaihan Krippendorff, founder of Outthinker Networks.
Fellow peers from the industry echo the sentiment. “We are seeing several enterprises pushing for these solutions. The reason is that Edge AI brings actionable intelligence and real-time decision-making possibilities into operational technology environments, such as the factory floor,” says Paul Bloudoff, senior director of edge services at NTT.
As such, the Edge AI market is rapidly expanding. Big names are joining the segment and are offering their own solutions. Among them are NTT Data, Siemens, Microsoft, and IBM. Each of these providers is focusing on a different idea. Siemens offers an industrial edge platform for manufacturers to use AI apps on the factory floor. IBM is focused on Edge AI solutions for healthcare, telecommunications and automotive. NTT Data develops a fully managed Edge AI solution which aims to “break down IT-OT silos, enabling advanced AI use-cases for industrial and manufacturing.”
LLMs like the Edge, too
LLMs (Large Language Models) are a niche of AI which is particularly hot right now. LLMs house the Generative AI projects like OpenAI’s ChatGPT, Microsoft Copilot, Google Gemini, MetaAI, etc. LLMs are not just chatbots, though. They can do a lot more tasks and features, depending on the way they are programmed and trained.
As such, LLMs are of big interest for enterprises, too. “By 2027, more than 50% of the GenAI models that enterprises use will be specific to either an industry or business function – up from approximately 1% in 2023. Domain models can be smaller, less computationally intensive, and lower the hallucination risks associated with general-purpose models,” Arun Chandrasekaran, an analyst at Gartner, told Data Center Knowledge.
That is great for business, but also highlights yet another big challenge for data centers; analysts say that for the foreseeable future, models running on the edge will continue to be trained in the data center. Thus, one of the main benefits of Edge AI – less traffic, won’t be fully realized as edge LLMs will constantly seek the data center for additional information, training and data.
This is why LLM developers have already started to work on streamlining and refining the models. We now have several versions which are much smaller, yet highly efficient. Most of them right now are focused for smartphones, bringing AI functions locally on the device. The next step towards LLMs specifically designed for Edge AI though is logical and will happen sooner rather than later.
It won’t be as easy as one would like
Of course, as with any new technology, it won’t be as easy as plugging it in and starting to enjoy the benefits immediately. There will be quite a few challenges in order to get things right. The 2023 Edge Advantage Report, for example, says that “nearly 40% of those planning edge deployments expressed concerns about the capabilities of their current infrastructure to support the technology.” And indeed, many enterprises are faced with obstacles they didn’t expect, says Bloudoff.
One of the main challenges is ensuring the data from IoT devices, sensors, machinery and other participants in the networks will be collected, processed and analyzed at the edge. This is where many enterprises find out that an Edge server doesn’t mean something small with modest hardware. Quite the opposite. It requires serious computing power which is able to handle big workloads with real-time expectations.
Also, it’s important to have a proper process to organize the gathering and processing of the data. Luckily, there are solutions being made in this area which will solve that part of the challenge. NTT for example is making an “ultralight edge AI platform” which has features for the management of AI applications at the edge. It also offers automation features for scanning the inventory assets, to identify potential vulnerabilities and more.
Is Edge AI secure enough?
Speaking of vulnerabilities, the natural question is how Edge AI measures up to the modern cyber security needs? AI and LLMs have their specific cyber security needs, which have to be further addressed in an edge setup. Especially if said Edge AI service is processing private data like health records, personal questions, company secrets, etc.
In some cases, Edge AI can actually be of benefit. If it’s able to handle the majority of queries locally and not transfer them to a data center, then there’s less for hackers to intercept. If the Edge AI setup is done by a service provider, then said entity will have a responsibility for the cyber security of the configuration. But if the enterprise has made the Edge setup within its own means, then the company itself will be responsible for keeping it secure and not relying on the defenses of the partnering data center or local security.
Edge AI is coming right now
Unlike other projects or ideas, Edge AI is already a fact and is being used in the real world. “We believe that the convergence between tower and cloud is something that has a long-term trajectory and will happen as the wireless operators start converting their networks to 5G and upgrading their Core As the cloud continues to grow, we see this need to have increasingly decentralized compute,” says John Rasweiler, SVP of innovation in the US tower division at American Tower to DataCenterDynamics.
The company is one of many which is already using edge servers, deploying them as colocation sites in the US. Mostly at base towers which are ideal for this. “Towers are the perfect location for micro data centers as real estate in cities is expensive and limited, hence it is very hard to build larger data centers here. Plus, they are already equipped with connectivity and power – two critical factors to enable data centers,” says STL Partners’ senior consultant Matt Bamforth in a blog post.
Edge AI is already improving operational efficiency in manufacturing, says Data Center Knowledge. For example, IoT Sensors on production machines are generating data which is instantly analyzed by Edge AI and predicts potential equipment failures. It flags the components which are about or could fail, thus helping the manufacturer to get on top of the maintenance. This reduces downtime and increases overall productivity.
Home appliance and electronics maker Haier is among the manufacturers already using Edge AI. Haier says this has led to optimized production processes and introduced the ability to customize products for local markets much more easily. The company is so happy with its Edge AI results it has even turned it into a business – COSMOPlat which offers integrated AI systems to other manufacturers.
Edge AI is obviously helping with smart cities, too. Several US cities are already using AI to improve traffic management on busy intersections. They are noting both less incidents and increased flow through rate at the smart intersections.
Edge AI is also bringing sustainability benefits. “By reducing energy consumption and network congestion, Edge AI is helping companies minimize their environmental impact while driving digital transformation,” says Bloudoff. So, as we can see, Edge AI is already bringing a lot of benefits to its users and it’s just getting started. Once it is developed even further on all fronts, it will be even more successful in improving AI services while bringing regular data centers a much-needed breath of fresh air via reduced workloads.